Listen to this story
|
The VP of Databricks and founder of MosaicML, Naveen Rao, is thrilled. Databricks today announced the launch of the world’s most powerful open-source model DBRX. This new model outperforms SOTA open-source models like Llama 2 70B, Mixtral-8x7B and Grok-1 across various benchmarks, including language understanding (MMLU), programming (Human Eval) and Math (GSM 8K).
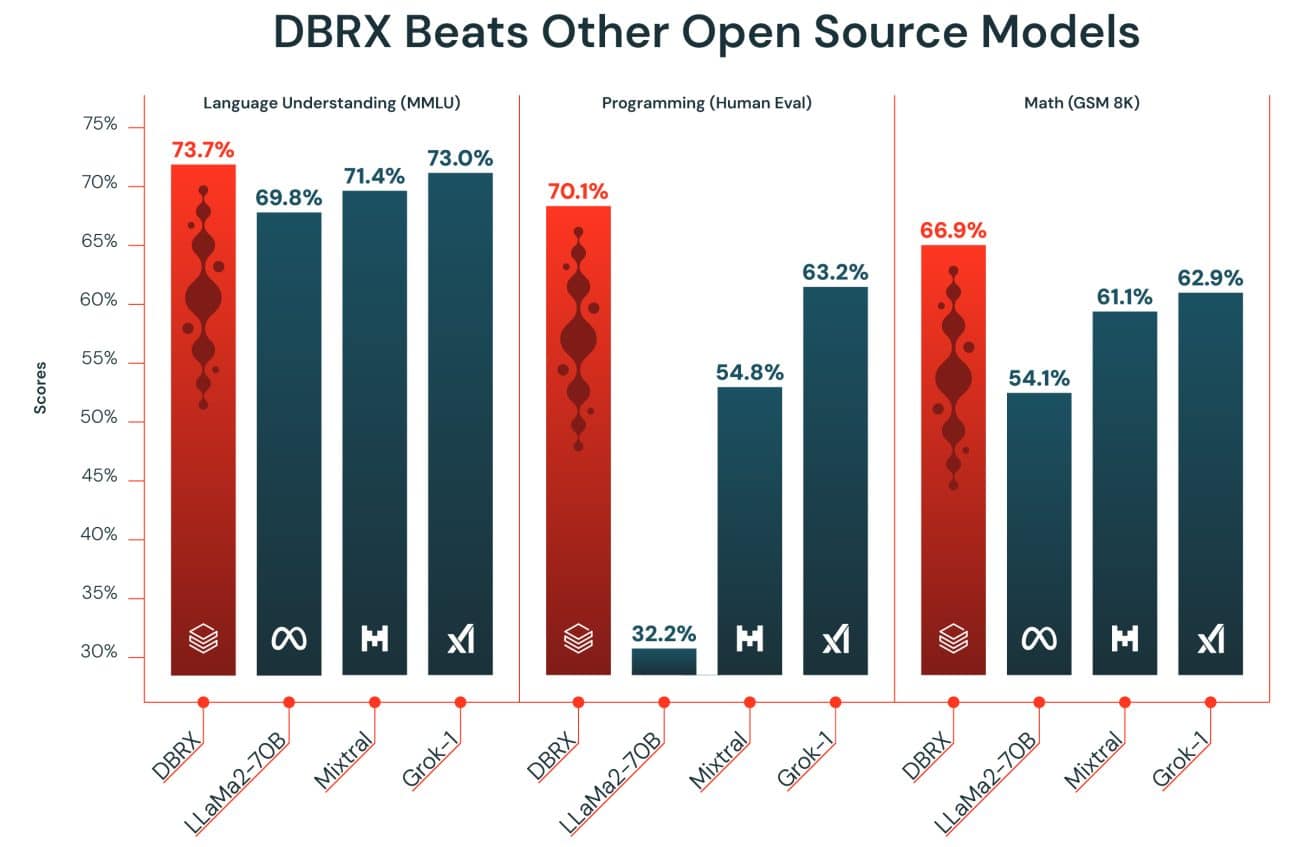
Interestingly, it also beat OpenAI’s GPT-3.5 and inches closer to GPT-4 on similar benchmarks, inevitably reducing the reliance on proprietary closed-source models with open-source models, and significantly reducing the cost.

“You can think of it as almost 2x better in some sense, but it’s also half the cost to serve it,” Rao said in an exclusive interaction with AIM ahead of the release. “It’s half the cost, half the flops, half the time,” he beamed.
Comparing its price with closed-source models like GPT-4, he said it is 1/10th of the dollars per token. Citing GPT-4, he said it is $120 per 1 million output tokens, whereas DBRX with a 32K context window is about $6.2 per 1 million token output—i.e., 20 times lower than that of GPT-4 for 1 million token outputs.
But, How?
This was possible because of the model’s MoE architecture, which provides significant economic benefits. “The economics are so much better for serving. They’re more than 2X better in terms of flops and floating point operations required to do the serving,” shared Rao.
It also enables fast performance, with DBRX outputting “100 tokens per second versus Llama putting out at 35 tokens per second”. Rao noted, “It comes out so fast that it almost looks like magic” compared to services like ChatGPT.
The 132B total parameter model has 36B “active parameters” due to the MoE design of having “16 separate models” where “when we make an inference, we choose a subset of four”, Rao said.
He also reiterated that while others have built MoE models, “we’re the ones who made it work, and at this scale. We’re not aware of anyone else who’s done it”.
The MoE architecture directly addresses key enterprise barriers like cost, privacy/control, and complexity that have hindered AI adoption, according to Rao.
Closes OpenAI’s Doors
Databricks mean business. Rao said that you can not beat OpenAI without a differentiated use case.
He said that to be successful, you need to outperform your competition. If you cannot offer a distinct advantage or a more cost-effective solution, there is no point in adopting someone else’s model. Simply trying to compete on equal terms is futile unless you can surpass them, said Rao.
He also said that it makes sense for Databricks to focus on helping enterprises build, train, and scale models catering to their specialised needs. “We care about enterprise adoption because that’s our business model. We make money when customers want to build, customise, and serve models,” he added.
Rao sees a turn in the tide with this model and senses that open-source models will eventually overtake closed ones like GPT-4, drawing parallels to Linux surpassing proprietary Unix systems.
“Open source is really just getting started. The world will look a bit different in five years,” said Rao.
Databricks is Not Alone
Recently, Elon Musk’s XAI open-sourced its LLM Grok-1 model for the ecosystem, which boasts 314 billion parameters. Rao wasn’t impressed. “I don’t think Grok 1 is that great, despite its large 314 billion parameter size,” he added, saying that it becomes difficult to evaluate as it takes a lot of compute.
He also pointed out that Grok’s capabilities are not commensurate with its massive scale, saying, “For a model that size, I would expect a higher level of capability.”
But, sadly that is not the case. He was rather dismissive of XAI’s Grok open-source model, its quality and capabilities relative to its massive scale, suggesting DBRX is a superior open-source alternative that outperforms Grok comprehensively on most benchmarks.
Meanwhile, Meta is also leading the open-source LLM race with Llama 2, and the release of Llama 3 on the horizon. Rao seems confident. He said that most of them would not be able to achieve the same economics. “I won’t be surprised if their model has the same economics as ours, but it is going to be of worse quality.”
He said that the key is to look at not just performance quality but also cost and time, like how fast it is, what it costs, and the quality, all put together. “When you look at these together, I don’t think anyone’s going to beat us in a long time,” said Rao, confidently.
Late to the Open Source LLM Party
The road to releasing its open-source model, DBRX, wasn’t smooth. Rao told AIM that Databricks wanted to release DBRX even earlier but faced challenges in getting the required compute resources and ensuring stability.
Rao mentioned that the release was “a month or two behind schedule” due to these issues. One of the biggest technical challenges was scaling up to the many required GPUs. Rao said, “We achieved the ability to scale to a large number of GPUs, but had to deal with some challenges along the way.”
He said acquiring a stable GPU cluster from their provider for training was a bottleneck, which involved “more than 3072 H100 GPUs” but their “provider was not super stable many of the times”.
Rao said that routing or selecting the models in the subset of four models (from the total of 16 models) within the MOE – mixture of experts architecture – was probably one of the biggest challenges to solve. “We’ve put considerable effort into engineering this system to optimise for efficiency and effectiveness,” said Rao.
He explained that an unstable “flaky” cluster led to slowdowns and failures. “When the cluster is flaky, things go slow or they break and stop working,” he said. But, he now seems hopeful and is more optimistic about building powerful open-source LLMs for enterprise customers and scaling them seamlessly.
What’s Next?
The launch demonstrates Databricks’ commitment to open source after acquiring generative AI startup MosaicML. Rao believes integrating its technology will enable companies to differentiate their AI and leverage proprietary data. The team looks forward to releasing new and better variants of the model.
“So RAG is a big, important pattern for us, and we’ll be releasing tools,” Rao revealed. Moreover, the innovation just doesn’t stop there. He said, “We already have them in private preview, but we have ways to do RAG within Databricks that are very straightforward and simple. Making this model the best generator model for RAG that’s important.”
Rao also said that the model is going to be hosted in their products on all the major cloud environments like AWS, GCP and Azure. “It is an open-source model, you can take it and serve it like you want,” Rao said.