Listen to this story
|
Data lakes have become an indispensable part of any modern data infrastructure due to their varied benefits. Owing to their ability to store large amounts of raw and unstructured data while providing democratic and secure access has made it a favourite of enterprises.
Estimates put the CAGR of the data lakes market at about 24.9%, with a predicted market size of $17.6 billion by 2026. With the bombastic growth that this market is seeing, it is no surprise that enterprises are finding new use cases for data lakes —— moving away from monolithic data lakes to domain-specific data lakes.
Why monolithic data lakes are bad
Data lakes undoubtedly offer benefits over the previous, more traditional approach of handling data, like ERP and CRM softwares. While the previous approach is more like small, self-owned, self-operated stores, data lakes can be compared to Walmart, where all the data can be found in a single place.
However, as the technology matures, enterprises are finding that this approach also comes with its set of drawbacks. Without proper management, large data lakes can quickly become data swamps — unmanageable pools of dirty data. In fact, there are 3 paradigms in which data lakes can fall apart, namely complexity, data quality, and security.
Flexibility is one of the biggest pros of maintaining a data lake, as they are large dumps of raw data in their native format. This data is also not stored in a hierarchical structure, instead using a flat architecture to store data.
However, with this flexibility also comes with added complexity, meaning that talented data scientists and engineers need to trawl through this data to derive value out of it. This cannot be done without specialised talent to maintain and operate it.
This leads into our next issue — data quality. Operating and sifting through a data lake consumes lots of time and resources, requiring constant data governance. If this is ignored, the data lake will become a data swamp, with lots of new data not being properly labelled or identified. Metadata is the key to a good data lake, and this requires constant governance.
Due to the centralised nature of data lakes, security becomes a concern with the number of teams that are accessing them. Access control is one of the most important facets of maintaining a data lake, as well as providing the right set of data to the right teams. If this is not done properly, sensitive data might become prone to leaks.
Even as data lakes have these drawbacks, their positive impact is undeniable. Their scalability, cost savings, and functionality are their biggest selling points. However, there is a way to get the best of both worlds — moving away from a monolithic data lake to various smaller, distributed data lakes.
A data monolith vs. a data mesh
As data lakes scale up, these issues have become more prominent, prompting companies to move to smaller, domain-specific data lakes. Termed as a data mesh, this is more of an organisational approach leveraging the benefits of data lakes with few of their drawbacks.
In a typical data lake, all of the organisation’s data is ingested into one platform, and then cleaned and transformed. This represents a move away from domain oriented data ownership to one that is more agnostic to the domains, creating a centralised monolith. Creating a data mesh bypasses these limitations, going back to domain oriented data ownership while maintaining the benefits of data lakes.
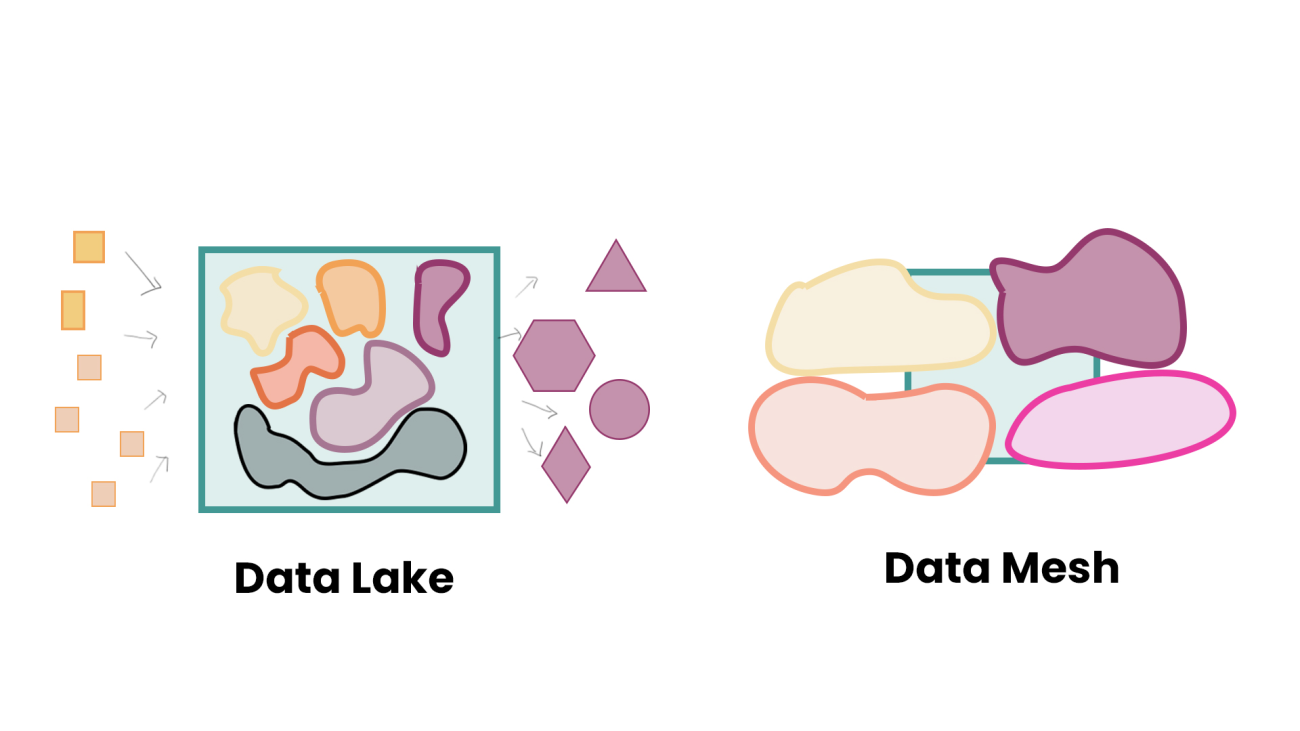
Instead of providing a centralised repository that various teams will access through access control, teams can take ownership of the data created in their domains. This approach not only reduces the amount of maintenance required for the overall data lake, but also gives democratised access to specific domains, allowing them to take charge of their data.
Data mesh bypasses the issues posed by monolithic data lakes. Data security becomes less of an issue when compared to a monolithic structure, as teams only access the data they need to, as opposed to controlled access to all the data. Complexity is also reduced, making it easier for data concierges to handle and manage the data.
Managing data quality also becomes easier, as the smaller the data lake is, the lesser the likelihood of it becoming a data swamp. However, even as smaller data lakes exist, it is important for them to be built upon an existing big data architecture to allow for cross-domain data access.
Even with the benefits data mesh offers, it is important to note that these benefits will only be seen as the data needs of a company scale up. At smaller scales, the benefits offered by data mesh will be outweighed by the benefits offered by a centralised data lake. As with any data architecture, companies must test what works for their specific use-cases.