There is rich and abundant data available in the telecom sector, and this data has been especially relevant in the last two years. Bandwidth consumption reached an all-time high amid the global health crisis, as all businesses and educational institutions moved towards a digital workspace model.
However, despite this shift to digital-first, some key challenges have led to a dip in growth in the sector. These challenges include:
- Intense pricing competition across the sector from both legacy players and newcomers offering unique business models.
- Increasing adoption of services from OTT providers (Ex: WhatsApp for voice call, Messenger for messaging, etc.) and,
- Raising capital expenditures to set up new infrastructure to provide improved connectivity and 5G services.
This article will discuss the top growth opportunities for the telecom sector in acquiring new customers while simultaneously growing the value of existing customers.
- Customer 360-view to enable targeted growth from the existing customer base.
- Customer retention.
- Customer service experience.
- Capitulating on the growth of the B2B segment.
Customer 360-view – Enhanced customer understanding
Customer 360-view, as the name suggests, is about the all-around picture. It provides a comprehensive understanding of customers by aggregating data from all touchpoints. This data traces the customer’s journey across various departments, all on one central platform.
We can further augment internal data sources with structured and unstructured external data sources, such as business profiles, demographic reports, social media data, etc. Unfortunately, this rich external data is usually stored in silos or never used.
Companies tend to shy away from adopting the Customer 360-view because of its challenges. One common one is the difference in entity names used in various internal systems and third-party data sources. Here is where implementing AI-based string matching algorithms has helped merge multiple disparate sources.
Similar to the example above, solutions can be found for companies struggling to implement the Customer 360-view because its advantages trump the challenges. Let’s look at some of the advantages:
- Unified view of customers across all departments — from business development to customer support.
- Scalable data that can be processed faster and at a minimal cost.
- Enabling AI and analytics-use cases (not exhaustive) such as:
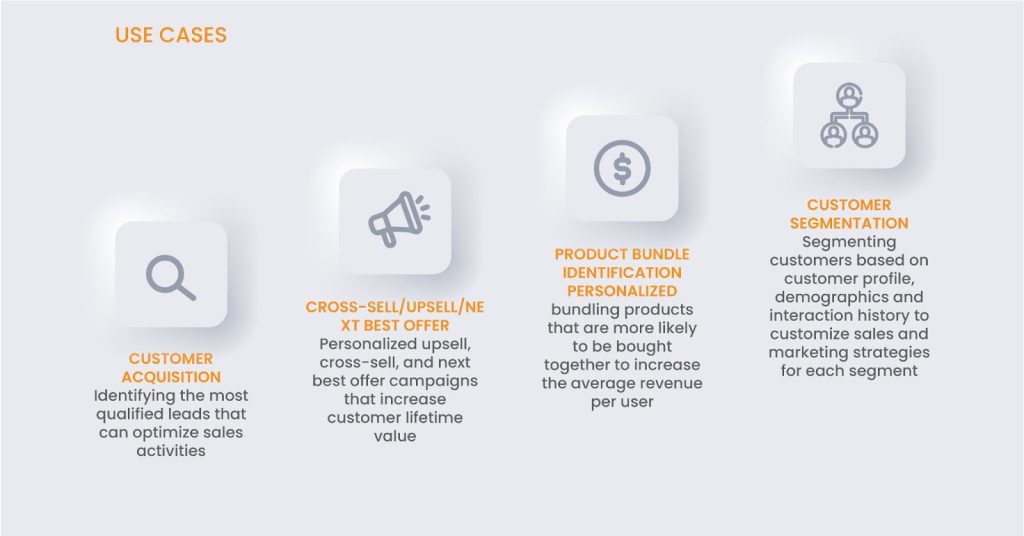
- Accurate external data augmentation has led to better features and thus improved accuracies in predictive models and improved understanding of customer behaviour.
Customer retention through churn prediction — The cost of customer retention is much lower than the cost of new customer acquisition
The offering of voice, messaging, mobile music, and video services by OTT producers such as WhatsApp, Messenger, Netflix, Spotify, etc., have made data the primary offering for telecom companies.
Due to the ongoing price wars and data-heavy plans with competitive pricing, customers are spoilt for choice. While the basic product is the same or has very few differences, the competition is high and has plenty of options. This has led to an increase in customer churn.
Hence, it is crucial for telecom companies to understand the reasons for this customer churn and predict paths that lead to an increase in customer churn.
One way to go about this is via machine-learning models that are able to predict customer churn. This can be done using customers’ past transactions, network quality, product pricing, product usage, customer feedback history, complaints log, demographics, and social media data (if any).
Targeting the right customers to carry out retention campaigns is key. Those picked will be directly related to the campaign budget, cost of retention of each customer, and the incremental revenue generated through each customer.
This process is especially important because even retaining a small percentage of the customers who are about to churn can lead to increased revenue impact in the long run.
Customer service transformation
If the offered products are similar and the competition is high, how does one differentiate between telecom operators? The answer is customer service. It is one of the key differentiating factors between companies.
In this digital-first world, there is an increasing demand for the transformation of customer experience and the adoption of new technology, such as AI-enabled chatbots and dialogue systems.
One common challenge is providing the customer with all the right information regarding their purchase product. Often, customer service officers handle a range of products and may not be equipped to handle all the customers’ questions. This increases the time customers spend on hold or in queues, which leads to dissatisfaction.
Here is where AI-enabled intelligent customer service systems can reduce waiting time and help in providing the most relevant solutions or recommendations to customers. This can be done in one or more ways:
- Forecasting inbound call volumes for optimizing short and long-term staffing and training.
- Virtual assistants to provide fast resolutions to all straightforward customer queries and redirect the rest to appropriate customer care agents
- Enabling the representative with a customer 360-view helps them understand the customer query and background without getting a lot of inputs from the customer.
- Enabling the team with a real-time analytics engine for recommending the right offer/product to an existing customer based on their profile, demographics, and interaction with the agent.
The growth of the telecom B2B segment to be driven by digitalization and 5G
The B2B business model enjoys high margins (compared to B2C), with customers willing to pay more for different services. It is characterized by a diverse list of products, customized solutions, pricing and multiple partners. On the downside, this increases the length of the sales cycle.
One common growth use case (apart from common telecom use cases discussed above) specific to the B2B segment is reducing the sales cycle time by using AI and analytics in product solutions and pricing. This leads to a better customer experience, thus increasing customer acquisition.
The following are the main differences in characteristics of the two segments:
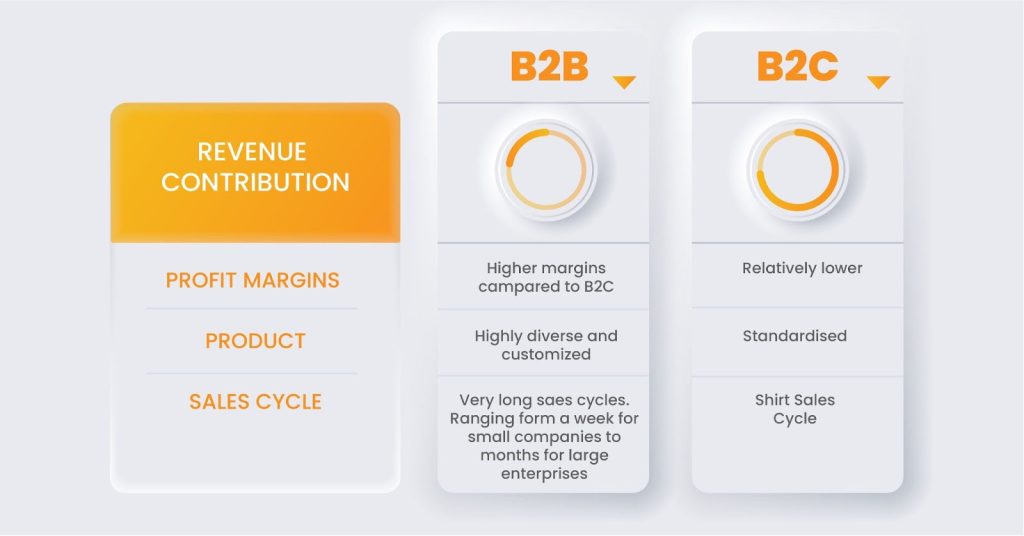
Historically, most telecom providers have prioritized analytics use cases to capture growth in the B2C segment¹. However, with the advent of digitalization, all businesses rely on the telecom industry for reliable high-speed 5G data and corporate mobile plans. It is estimated that by 2035, sales amounting to USD 13.2 trillion will be enabled by the 5G ecosystem ².
As a result, the next decade will likely see the B2B segment growing much faster than the B2C segment. As a result, concentrating on B2B use cases will help telecom companies grab a bigger share of the growing market.
Benefits of implementing AI and advanced analytics (examples)
To really understand how AI and analytics are helping transform this booming sector, let’s look at some real examples.
Customer 360 — Data governance system for an Asian OTT video service provider
Problem: The client was looking forward to developing a comprehensive understanding of the user’s program viewing behaviour for smarter programming and advertising decisions.
Solution: The solution was to build a data lake to process internal and third-party data from structured and unstructured data sources. Some key challenges included creating a data governance process, handling inconsistencies across multiple sources, and building a flexible system that allows new data sources.
Value delivered: The outcome of the exercise was a data lake that could process 100 GB of data volume daily with varying velocities, ensuring data availability for data analytics projects across multiple themes.
The following are select case studies executed using Customer 360-view datasets:

Churn prediction – User behaviour prediction model driving USD 4.5 MM annual revenue
Problem: The client, a telecom giant, wanted to identify customers most likely to churn in their video-on-demand (VOD) business.
Solution: The key challenges were huge data volume, limited metadata on VOD content, constantly changing user base, and limited subscriber demographic information. The solution involved building a random forest-based churn classification model based on the features extracted from past customer RFM behaviour, rate of change in purchases month-on-month, demographics, and content metadata.
Value delivered: A total of 73.4 % of potential churners were captured by the top 50% of the population flagged off by the model, leading to revenue retention of up to USD 4.5 MM per annum.
Customer service transformation case studies

Telecom B2B – Pricing system for a leading Asian telecom company.
Problem: The client was looking to shorten their B2B product sales cycle, which currently took up to 4+ weeks to produce the initial quotation.
Solution: The bottleneck in the process was identified as the involvement of third-party costs and the delay in receiving them. The solution involved building ML models in predicting third-party expenses to reduce the waiting time and provide customers with an initial quote.
Value delivered: The business impact was reduced turnaround time for an initial quote from four weeks to a maximum of one day.
The future is brighter, smarter, quicker
The applications of AI and predictive analytics in the telecom sector are endless. With digital transformation being the key goal for any company today, combining AI and analytics can not only help in delivering superior performance but also give a company that touch of uniqueness needed to survive in a cut-throat market.
For more information on services and use cases, please get in touch with us at https://www.tigeranalytics.com/.