Stanford University recently released the 2021 AI Index, highlighting major trends and advancements in artificial intelligence. The fourth edition of the report talked about technology’s impact on society, education, and policy and outlined the progress made in other AI subdomains such as deep learning, object detection, NLP, etc.
The highlights from the 2021 report included AI research citations, AI startup fundings, and growing conversation around AI ethics. One of the more significant observations made in the report was about the need for more and better benchmarks in AI and other related fields such as ethics, NLP, and computer vision.
“We’re running out of tests as fast as we can build them,” said Jack Clark, head of an OECD group working on algorithm impact assessment and former policy director for OpenAI.
What Are Benchmarks?
Benchmarks check the worthiness of a system to be deployed for real-time situations. They provide a reliable, transparent, standardised approach to gauge performance with different parameters for handling a workload.
So while a task and the metrics associated with a model can be thought of as an abstraction of the problem at hand, benchmark datasets provide fixed representations of tasks to be solved by a model.
Benchmarking is an important driver for research and innovation. Experts such as David Patterson, author of Computer Architecture: A Qualitative Approach, believe that good benchmarks help researchers compare ideas quickly, which results in better innovation.
Growing Need For Better Benchmarks
Research and development in AI are happening at lightning speed. As a result, benchmarks are getting saturated quickly. For instance, new models are released every month in NLP, and the previously held benchmark falls short, leading to overfitting.
However, the good news is that the open-source movement and increased collaboration between the researchers’ community have led to better AI/ML benchmarks.
A good benchmark has several purposes.
- For beginners, benchmarks help in sailing through new terms and data.
- For experienced researchers, benchmarks offer a quick-to-collect baseline. Any disagreement between the benchmark and specific measurements of the model can help identify areas of improvement.
- For users and solution providers, benchmarks help in estimating the developmental costs of infrastructure.
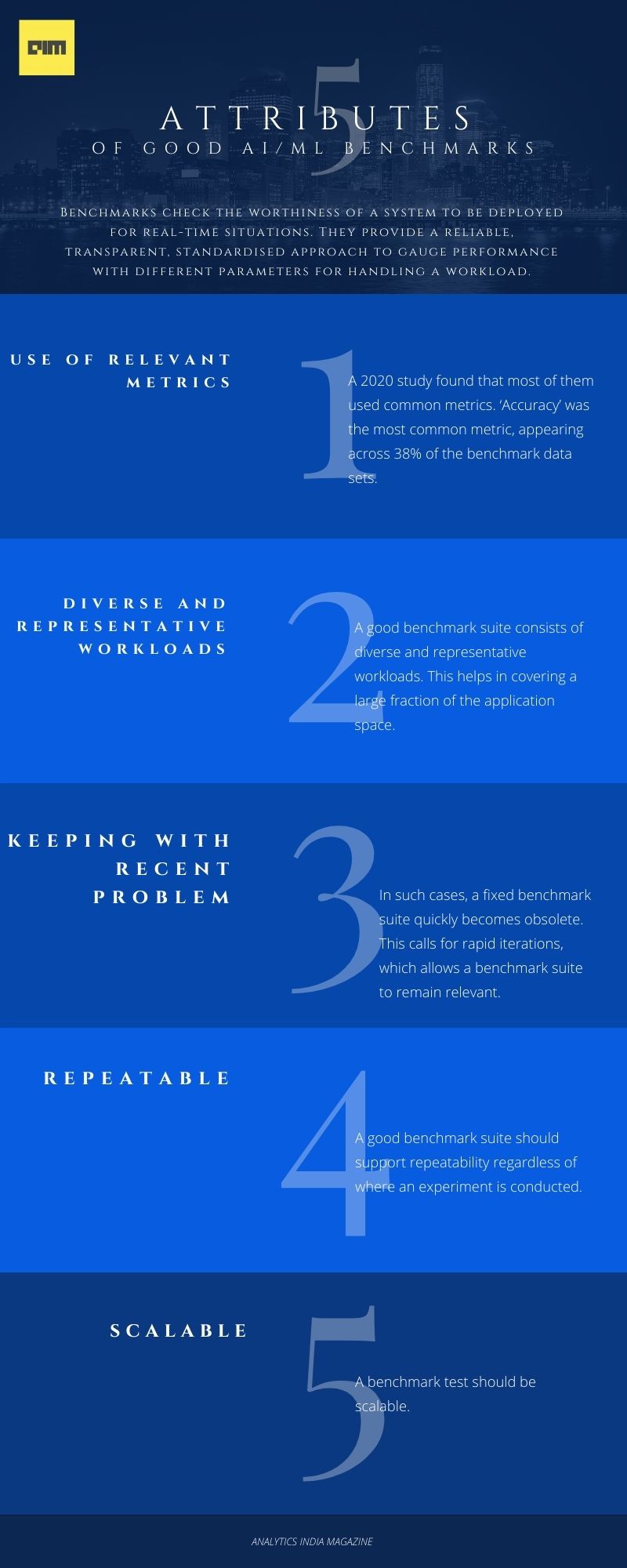
Representative benchmarks allow engineering efforts to be focussed on high-value and widely used targets. Benchmarks help optimise the system and ensure improved value and RoI for all the stakeholders–manufacturers, users, researchers, consultants, and analysts.
The attributes of good AI/ML benchmarks:
- The use of relevant metrics is critical. A 2020 study conducted on 3,000 research papers available on Papers with Code found that most of them used common metrics. ‘Accuracy’ was the most common metric, appearing across 38 percent of the benchmark data sets. The drawback with this is that the results could be uninformative, unuseful, and sometimes irrelevant.
- A good benchmark suite consists of diverse and representative workloads. This helps in covering a large fraction of the application space.
- The benchmarks chosen should be in keeping with the recent problem. In such cases, a fixed benchmark suite quickly becomes obsolete. This calls for rapid iterations, which allows a benchmark suite to remain relevant.
- A good benchmark suite should support repeatability regardless of where an experiment is conducted.
- A benchmark test should be scalable.
AI Ethics & Benchmarks
The 2021 AI Index also noted that despite the growing conversation around AI ethics and related domains, the field significantly lacks benchmarks to measure or assess relationships between technologies and their impact on society. Citing an example of a study by the National Institute of Standards and Technology on facial recognition performance focusing on bias, the report said while it is a challenge to create more data and relevant benchmarks, it is still an important area to focus on. “Policymakers are keenly aware of ethical concerns pertaining to AI, but it is easier for them to manage what they can measure, so finding ways to translate qualitative arguments into quantitative data is an essential step in the process,” the report stated.