Listen to this story
|
In the recent past, it is no secret that AI, especially cutting-edge deep learning algorithms, have been plagued by the explainability crisis. In May 2021, two researchers from computer scientist Su-In Lee’s Lab of Explainable AI for Biological and Medical Sciences in Seattle published a paper in Nature titled, ‘AI for radiographic COVID-19 detection selects shortcuts over signal’, discussing the far-reaching effects of this issue.
With the increased usage of AI in critical industries like healthcare and finance, explainability has become even more critical. In the heyday of the COVID-19 crisis, researchers started developing AI systems to detect COVID-19 accurately. But the technique that the systems used remained behind the curtain. When tested in labs, these systems worked perfectly but when used in hospitals, they failed. The paper explained how to use AI successfully in medical imaging, it was essential to crack the ‘black box’ in ML models.
Models have to be trustworthy and interpretable. This was all well and good. Until explainable AI became a marketing point for most companies selling these AI systems. However, it seemed like AI didn’t just have an explainability problem, explainability itself had a problem.
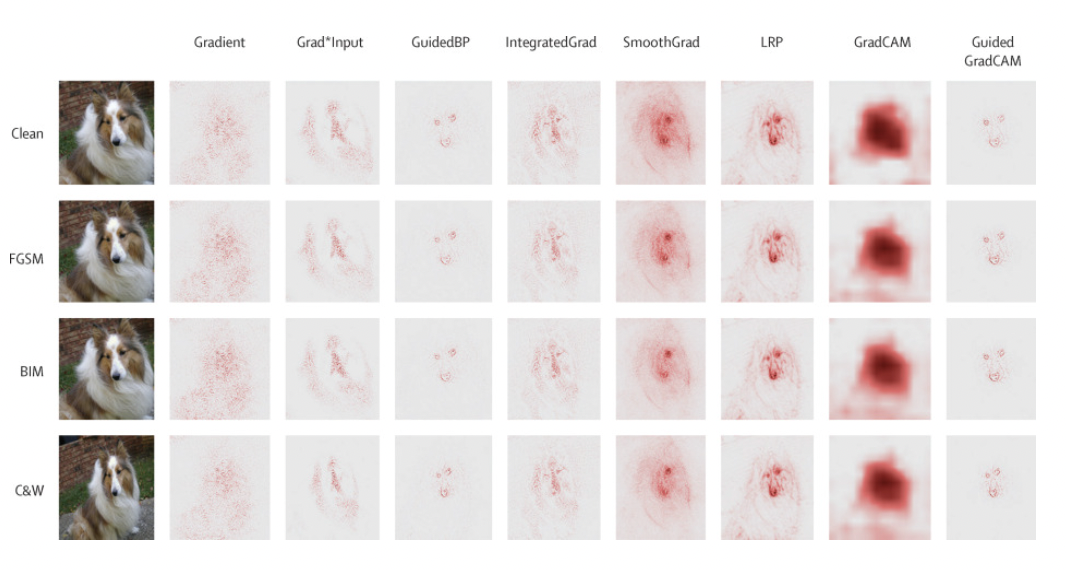
Current problems with explainability techniques
Last year in November, Lancet Digital Health published a paper by MIT computer scientist Marzyeh Ghassemi claiming that explainable AI itself couldn’t be understood easily. Current explainability techniques were only able to produce “broad descriptions of how the AI system works in a general sense” but when asked to justify how individual decisions were made, the explanations were “unreliable and superficial”.
The paper goes on to use another commonly used explainability method called ‘saliency maps’ as an example. These maps produce a heat map on an image of the algorithm and highlight the parts that were most reliant on AI for a prediction. The paper demonstrates how one heat map came to the conclusion that the patient had pneumonia but couldn’t reason why it thought so.
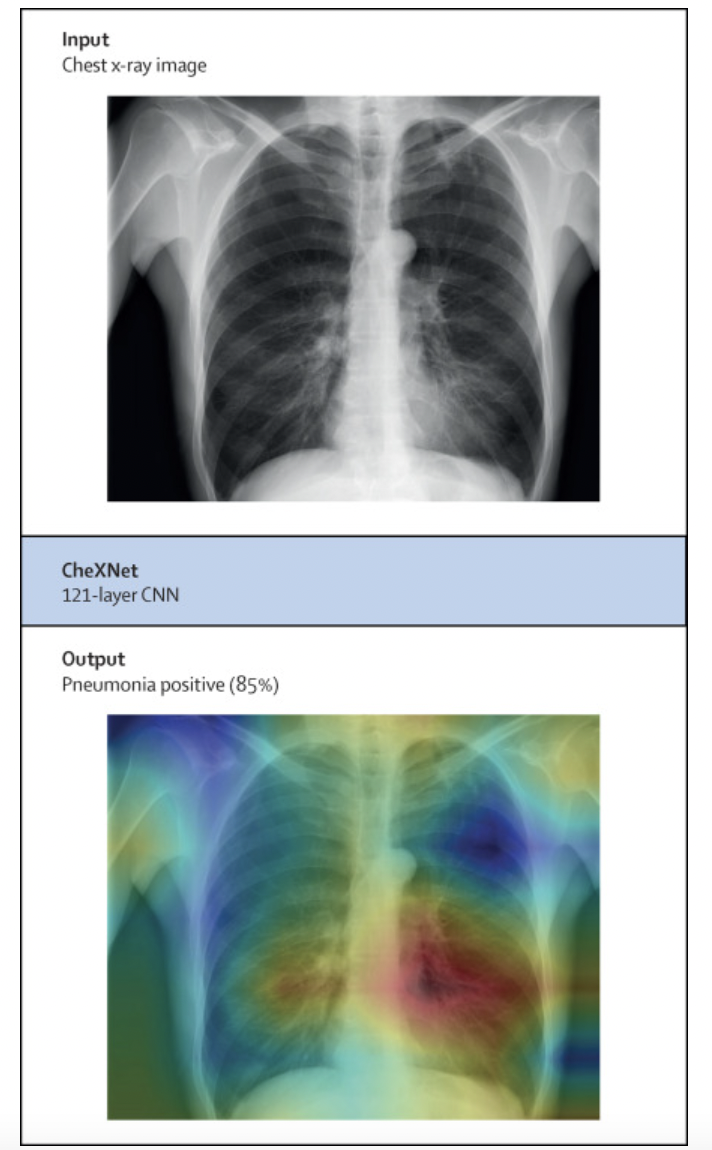
Interpretable models not Explainable AI
Experts from other sectors too have asked for explainable AI to be looked at from a different perspective. Agus Sudjianto, executive vice president and head of corporate model risk at Wells Fargo, put it this way, “What we need is interpretable and not explainable machine learning.”
Sudjianto fully acknowledges that models can fail and when they fail in a sector as vital and directly impactful as banking, they can have serious consequences on a client. For instance, someone in an urgent need of a loan might have their request rejected.
“This is a challenging task for complex machine learning models and having an explainable model is a key enabler. Machine learning explainability has become an active area of academic research and an industry in its own right. Despite all the progress that has been made, machine learning explainers are still fraught with weakness and complexity,” he stated. Sudjianto goes on to argue that what ML needed in this case was interpretable ML models that are self-explanatory.

A new approach
In a paper co-authored by Wells Fargo’s Linwei Hu, Jie Chen, and Vijayan N. Nair, titled, “Using Model-Based Trees with Boosting to Fit Low-Order Functional ANOVA Models,” a new approach to solving the interpretability problem was proposed.
The earliest solutions to interpretability were all post hoc and included low-dimensional summaries for high-dimensional models that were complex. These were ultimately incapable of giving a full bodied picture. The second solution given for model interpretability was using surrogate models to fit simpler models to draw information from the original models which were complex.
Examples of these models are LIME—first proposed in a 2016 paper by Marco Tulio Riberio—which is based on linear models and offers local explanations; and locally additive trees for global explanation.
Another new path forward was to use ML algorithms to fit these easily interpretable models that are extensions of generalised additive models (GAMs). The logic behind using these algorithms was to slow down the usage of normally complex algorithms for large-scale applications with pattern recognition and instead use nonparametric models with low-order that were able to figure out the structure.
Another paper co-authored by Sudjianto explained why inherently Interpretable machine learning (IML) models should be adopted due to their transparency rather than black-boxes as model-agnostic explainability isn’t really easily explained or defended under scrutiny from regulators.
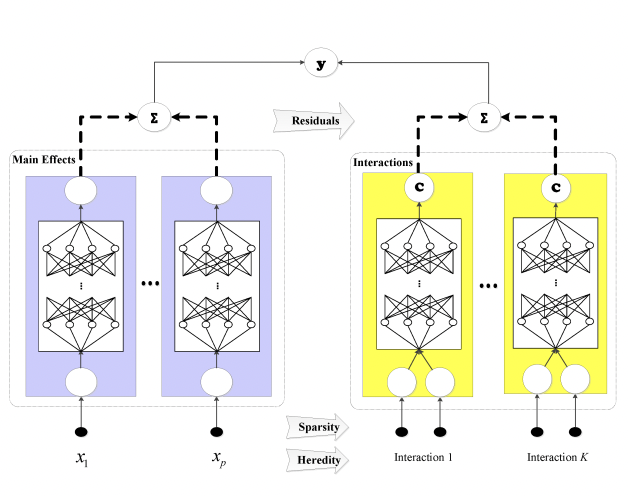
interactions, b) heredity on the candidate pairwise interactions; and c) marginal clarity to avoid confusion between
main effects and their child pairwise interactions, Source: Research
The study suggested a qualitative template based on the feature effects and the constraints of the model architecture. The study listed the design principles for the development of high quality IML models, with examples from tests done on ExNN, GAMI-Net, SIMTree, and so on. Eventually, these models were proven to have greater practical application in high-risk industries such as the financial sector.
So, has AI explainability largely become a fraudulent practice? Sudjianto seems to think so. “There is a lack of understanding of the weaknesses and limitations of post hoc explainability which is a problem. There are parties who promote the practice who are less than honest, which has misled users. Since the democratisation of the explainability tools, matters have become worse because users are not trained and not aware about the problem,” he stated.
Sudjianto also believes that the problem lies both with the concept of explainable AI as well as the prevalent techniques. “Post hoc explainer tools are not trustworthy. The techniques give a false sense of security. For high risk applications, people should design in interpretability,” he added.