There are around 1500 active volcanoes worldwide, and more than 80 erupt each year. These eruptions occur with no warning leading to irrecoverable loss of property and life.
Forecasting an eruption requires round the clock monitoring along with a fool proof system. The cost of maintaining such systems is high. Not to forget the abrupt nature of these eruptions. This usually forces the authorities to settle for ground-based sensors.
This makes the monitoring and warning difficult in active zones.
To address this issue, a team led by Sébastien Valade from the Technical University of Berlin (TU Berlin) and the GFZ German Research Centre for Geosciences in Potsdam develop a new volcano monitoring platform that analyses satellite images using artificial intelligence (AI).
“Apart from seismicity, all of these can be monitored from space by exploiting various wavelengths across the electromagnetic spectrum,” wrote Sébastien Valade, leader of the MOUNT project, in their paper.
The team behind MOUNT(Monitoring Unrest from Space) tried to build a warning system with less false positives by integrating multiple sets of diverse data types for comprehensive monitoring of volcanoes.
MOUNTS – An Early Warning System
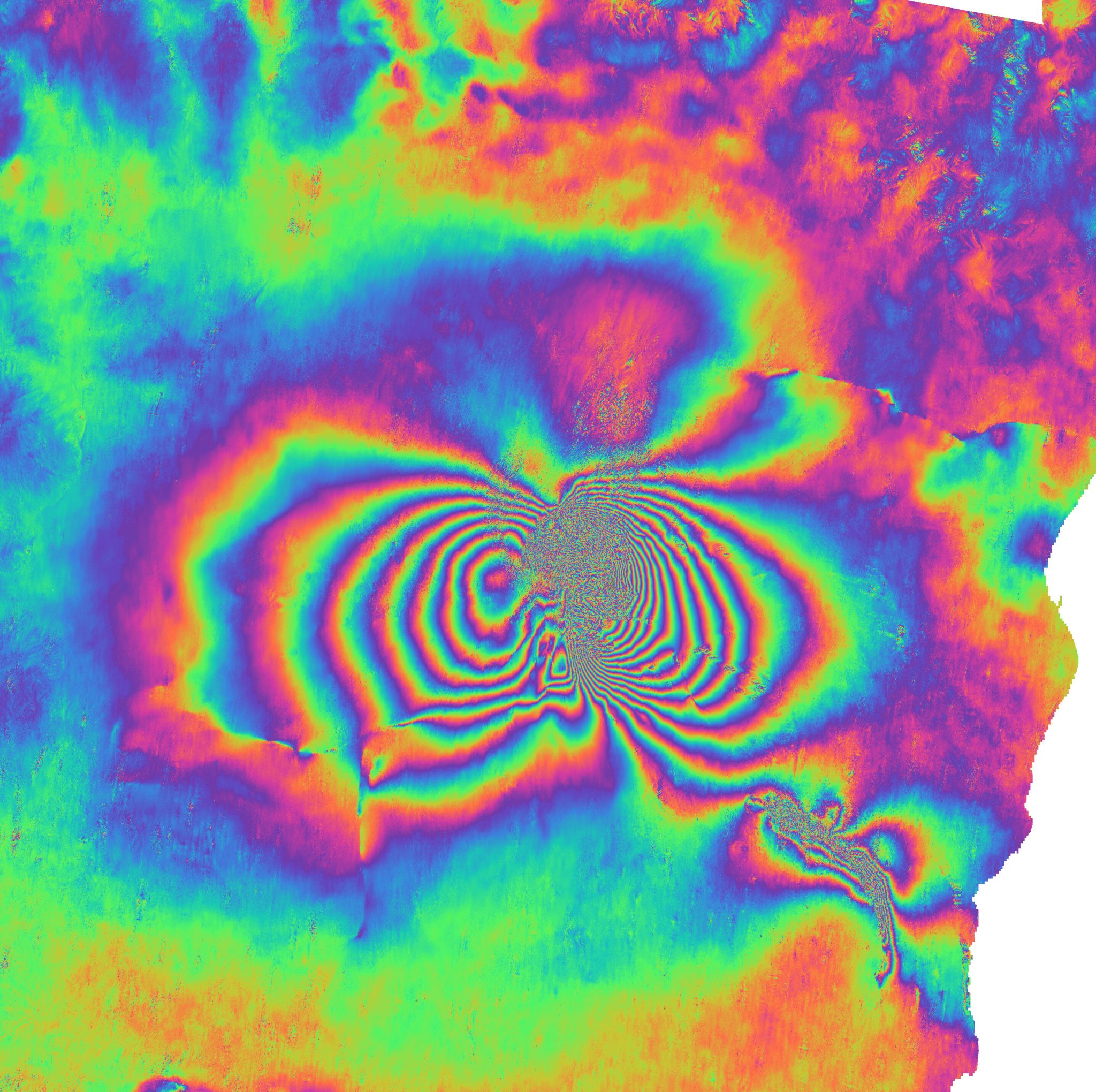
Land deformation and displacement are one of the few characteristics of an erupting volcano. So, if a system can be made to spot these changes, then a robust warning system can be built.
Since AI systems, especially the computer vision algorithms, have been improving at a tremendous pace, there can’t be a better option than the use of state-of-the-art models like convolutional neural networks(CNNs).
The deformation detection is performed using artificial intelligence, which recovers filtered phase gradients in wrapped geocoded interferograms. Interferograms spatially map ground surface movements.
As can be seen in the above picture, an Interferogram of the December 2018 eruption of Etna in southern Italy, based on Sentinel-1 satellite images.
The main challenges Valade and his co-authors had to deal with were handling the large amounts of data, and software engineering issues.
A pre-trained Convolutional Neural Network (CNN) can be incorporated into the processing pipeline to detect large deformation in InSAR interferograms (e.g., related to dyke intrusions), in an automated, timely, and robust fashion.
The network was designed as a fully convolutional auto encoder built out of residual learning blocks.
Once trained, the network is plugged into the processing pipeline newly generated interferograms are fed through this pre-trained network, which yields both the phase gradients and phase decorrelation (i.e., noise mask). Unwrapping of these phase gradients is then performed to recover approximate ground displacements.
CNN performs the filtering of the gradients and detection of noise, treatment of decorrellated areas. From the displacement map a deformation score is ultimately computed, as the standard deviation of the displacements.
The researchers successfully tested MOUNTS on a number of recent events like the Krakatau eruption in Indonesia in 2018 or eruptions in Hawaii and Guatemala, to name a few.
The system currently monitors 17 volcanoes worldwide including the Popocatépetl in Mexico and Etna in Italy.
Concluding Thoughts
Around the world, about 800 million people live within 100 kilometers of a volcano, posing a threat to lives and livelihoods. More than half of the world’s active volcanoes are not monitored instrumentally. Even eruptions that occur in remote areas can spread ash and disrupt public health, agriculture and economies.
Once the eruption starts on the other hand, the key to decipher the eruptive dynamics and to mitigate the related hazards, is to integrate multiparametric dataset streaming from both space- and ground-based sensors, in order to provide the most comprehensive view of the eruptive phenomena. Both require “monitoring”,over long periods of time, during both quiescent and eruptive phases.
Know more about the experiment here