Listen to this story
|
The amount of scientific research in AI has been growing exponentially over the last few years, making it challenging for scientists and practitioners to keep track of the progress. Reports suggest that the number of ML papers doubles every 23 months. One of the reasons behind it is that AI is being leveraged in diverse disciplines like mathematics, statistics, physics, medicine, and biochemistry. This poses a unique challenge of organising different ideas and understanding new scientific connections.
To this end, a group of researchers led by Mario Krenn and others from the Max Planck Institute for the Science of Light (MPL), Erlangen, Germany, the University of California, the University of Toronto, etc., jointly released a study on high-quality link prediction in an exponentially growing knowledge network. The paper is titled—‘Predicting the Future of AI with AI’.
Predicting future of AI research
The motivation behind this research was to envision a programme that could ‘read, comprehend, and act’ on AI-related literature. Being able to do so will open doors to predict and suggest research ideas which traverse cross-domain boundaries. The team believes that this will improve the productivity of AI researchers in the longer run, open up newer avenues of research, and guide progress in the field.
New research ideas, more often than not, emerge by making novel connections between seemingly unrelated topics/domains. This motivated the team to formulate the evolution of AI literature as a temporal network modelling task. The team has created an evolving semantic network that characterises the content and evolution of AI literature since 1994. To this end, they investigated a network that contained 64,000 concepts, also called nodes, and 18 million edges connecting two concepts. The team used the semantic network as an input to ten diverse statistical and machine learning methods.
One of the most foundational tasks—building a semantic network—helps in extracting knowledge from it and subsequently process using computer algorithms. At first, the team considered using large language models like GPT-3 and PaLM for creating such a network. However, the major challenge was that these models still struggled with reasoning, making it difficult to identify or suggest new concept combinations.

They then moved on to an approach borrowed from biochemistry, where knowledge networks are created from co-occurring concepts in scientific papers; individual biomolecules represent a node, and two nodes are linked when a paper mentions two corresponding biomolecules. This method was first introduced by Andrey Rzhetsky and team.
Using this method for progress in AI allowed the team to capture the history of the field. Non-trivial statements about the collective behaviour of scientists were extracted using supercomputer simulations, and the process was repeated for a large dataset of papers resulting in a network that captures actionable content.
The team developed a new benchmark called Science4Cast on real-world graphs. Following this, they provided ten diverse methods to solve the benchmark. Doing so helped in building a tool that suggests meaningful research directions in AI.
Read the full paper here.
Keeping up with AI research
The problem that the team set out to solve is one of the most discussed among AI academicians and practitioners. “The times are slightly neurotic,” said Cliff Young, a Google software engineer in a keynote speech at the Linley Group Fall Processor Conference in 2018. He added that AI had reached an exponential phase at the same time as Moore’s Law came to a standstill.
The same holds true even four years later. In fact, the progress in AI research might have even paced up given the rapid digitalisation that happened during the global pandemic. Just take this year for example. It started with OpenAI rolling out DALL.E 2 which gave way to other text-to-image generation tools, each better than the previous one. It has not been even a year and we have other similar text-to-X tools—think text-to-video, text-to-3D image, and even text-to-audio.
There are some decade old challenges that AI has managed to solve. Called the 50-year-old grand challenge, it was a mystery for the scientific community to understand how proteins fold up. But with DeepMind’s AlphaFold, scientists could get protein structure predictions for nearly all catalogued proteins. To fully appreciate the innovation, we must understand that in 1969 American biologist Cyrus Levinthal hypothesised that it would take longer than the age of the known universe to enumerate all possible configurations of a typical protein by brute force calculation.
Very recently, DeepMind introduced AlphaTensor, an AI system to discover correct algorithms for performing tasks such as matrix multiplication. This solves a 50-year-old open question in mathematics about finding the fastest way to multiply two matrices.

The sheer magnitude and pace at which AI research is happening can be overwhelming. Open any AI and machine learning-related forum, and you will find ‘keeping up with AI progress’ as the top topic of discussion. Hopefully, the research by Krenn et al. will ease some pressure.
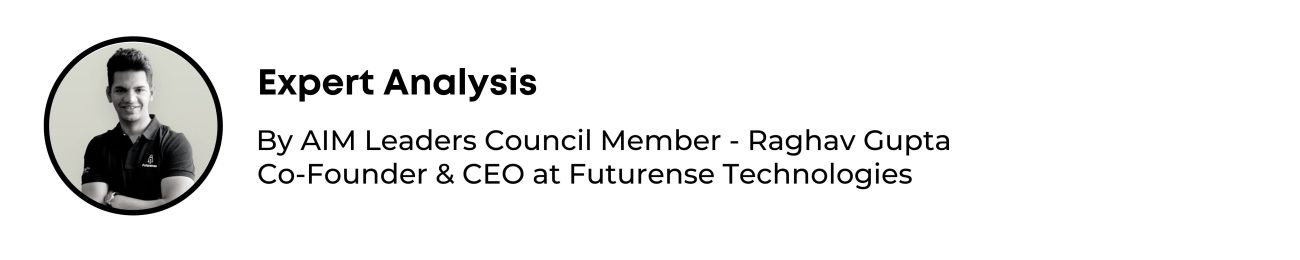
I feel the article advocates the long-drawn question of the world-renowned computer scientist – Alan Turing – Can machines think? Well, it turns out they might! I feel an upending and high-quality prediction tool/programme is highly critical to enable personalized AI-research direction to accelerate AI’s progress and its use case in an exponentially growing knowledge network environment. I really appreciate for turning the spotlight on the critical role of algorithms and the evolution of various models from semantic networks to biomolecules that represented knowledge in a simpler, comprehensible, and transparent manner. On the contrary, language shares a deep connection with human societies and they become the grail of AI research also depends on the full grasp of the human language and its nuances.
Despite the challenges expressed by the scientific community in leveraging AI for meaningful research directions, I see powerful methods with a carefully curated set of network features can set the tone for unleashing the potential of AI with correct algorithms in driving better predictions without human intelligence and advancing scientific research and ideas.