Facebook AI researchers Ruohan Gao and Kristen Grauman have introduced VisualVoice, a new approach for audio-visual speech separation.
“Whereas existing methods focus on learning the alignment between the speaker’s lip movements and the sounds they generate, we propose to leverage the speaker’s face appearance as an additional prior to isolate the corresponding vocal qualities they are likely to produce,” the researchers said.
The human perceptual system draws heavily on visual information to reduce ambiguities in the audio and modulate attention on an active speaker in a busy environment. Automating this process of speech separation has many valuable applications, including:
- Assistive technology for the hearing impaired.
- Superhuman hearing in a wearable augmented reality device.
- Better transcription of spoken content in noisy in-the-wild internet videos.
Procedure
The researchers first formally defined the problem before presenting the audio-visual speech separation network. The paper then introduced learning audiovisual speech separation and cross-modal face-voice embeddings in a multi-task learning framework and finally presented the training criteria and inference procedures
“We use the visual cues in the face track to guide the speech separation for each speaker. The visual stream of our network consists of two parts: a lip motion analysis network and a facial attributes analysis network,” the paper read.
The lip motion analysis network includes:
- The lip motion analysis network takes N mouth regions of interest (ROIs) as input, and it consists of a 3D convolutional layer.
- This is followed by a ShuffleNet v2 network to extract a time-indexed sequence of feature vectors.
- They are then processed by a temporal convolutional network (TCN) to extract the final lip motion feature map of dimension Vl×N.
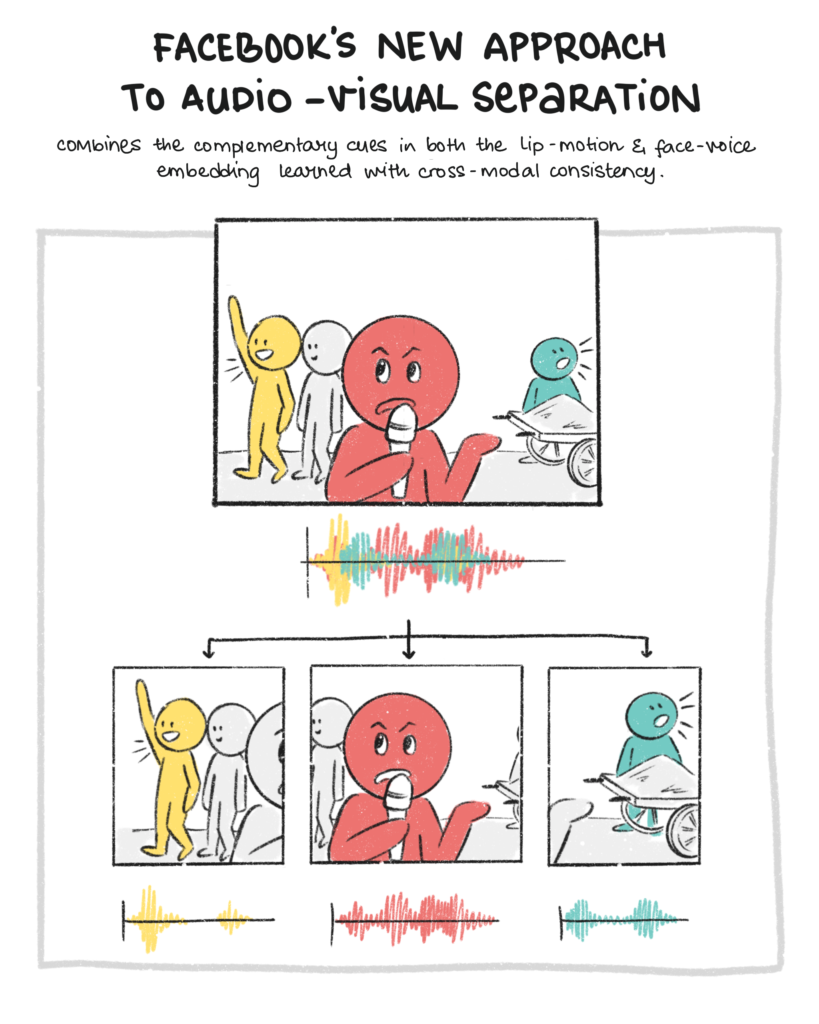
For the facial attributes analysis network, researchers used:
- ResNet-18 network that takes a single face image randomly sampled from the face track as input to extract a face embedding that encodes the facial attributes of the speaker.
- Then replicate the facial attributes feature along the time dimension to concatenate with the lip motion feature map and obtain a final visual feature.
“The facial attributes feature represents an identity code whose role is to identify the space of expected frequencies or other audio properties for the speaker’s voice, while the role of the lip motion is to isolate the articulated speech specific to that segment. Together they provide complementary visual cues to guide the speech separation process.”
On the audio side, the team uses a U-Net style network tailored to audio-visual speech separation. It consists of an encoder and a decoder network.
Result & scope
Lip motion is directly correlated with the speech content and is much more informative for speech separation. However, the performance of the lip motion-based model suffers dramatically when the lip motion is unreliable, as often the case in real-world videos. “Our VISUALVOICE approach combines the complementary cues in both the lip motion and the face-voice embedding learned with cross-modal consistency and thus is less vulnerable to unreliable lip motion,” said researchers. Check out the demo here.
Cross-modal embedding learning could benefit from the Facebook model’s joint learning. Researchers intend to evaluate the cross-modal verification task, in which the system must decide if a given face and voice belong to the same person.
“Our design for the cross-modal matching and speaker consistency losses is not restricted to the speech separation task and can be potentially useful for other audio-visual applications, such as learning intermediate features for speaker identification and sound source localization. As future work, we plan to explicitly model the fine-grained cross-modal attributes of faces and voices and leverage them to further enhance speech separation,” the researchers concluded.