Google DeepMind is making sure to leverage AI to create a tangible impact, be it with AlphaFold for protein folding or GNoMe for material science. Taking a step further, the R&D arm of Alphabet, introduced AlphaGeometry, an AI system that solves complex geometry problems at a level approaching a human Olympiad gold-medalist. The AlphaGeometry code and model is open sourced, as well.
While the previous state-of-the-art system called Wu’s method solved 10 of 30 Olympiad questions, the new model solved 25 geometry problems, within the competition time frame. An average human gold medalist solved 25.9 problems.
Training Method
AlphaGeometry addresses the challenges faced by AI systems in solving complicated geometry and mathematics problems by combining a neural language model with a rule-based deduction engine. This neuro-symbolic system generates 100 million synthetic training examples, sidestepping the need for human demonstrations and overcoming data limitations.

Alongside other synthetic data generation and training methods, AlphaGeometry aims to unlock new possibilities in mathematics, science, and AI. Operating on a “thinking, fast and slow” approach, the neural model provides quick, intuitive ideas, while the deduction engine ensures deliberate, rational decision-making.
Synthetic Data Generation
To train AlphaGeometry without relying on human demonstrations, the team used a synthetic data generation method that mimics the human process of learning geometry at scale. Using highly parallelized computing, the system generated one billion random geometric diagrams, establishing exhaustive relationships between points and lines in each diagram.
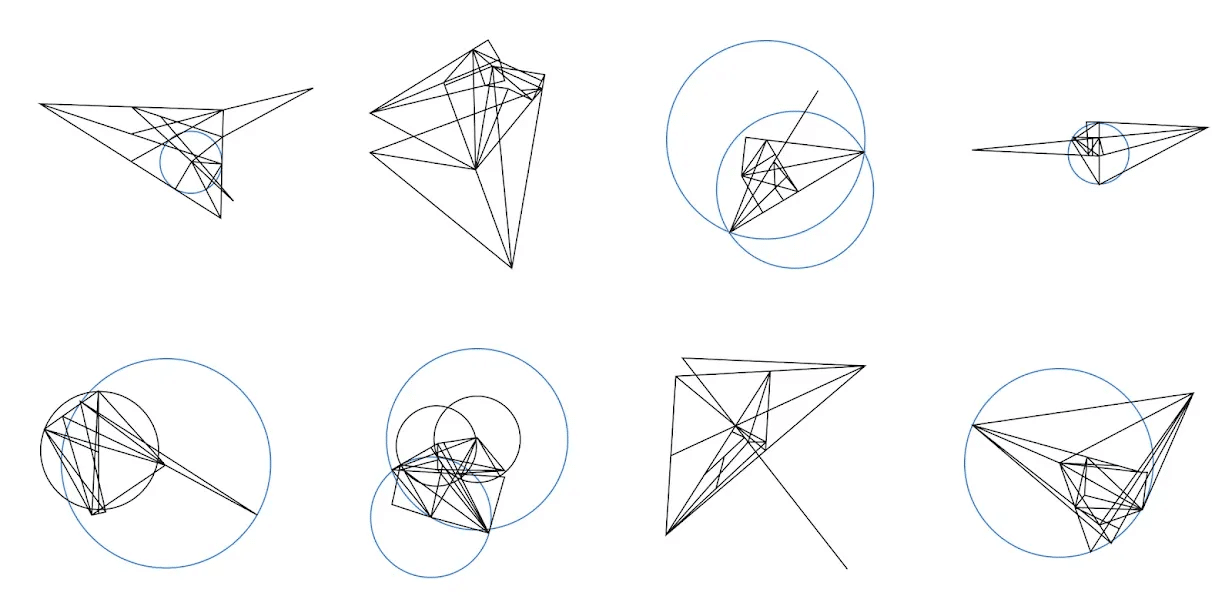
Through a process called “symbolic deduction and traceback,” AlphaGeometry identified proofs within each diagram and determined the additional constructs needed. The resulting dataset was refined to 100 million unique examples, encompassing varying difficulty levels, with nine million featuring added constructs.