Listen to this story
|
Creativity has taken a new form and shape thanks to the advent of text-to-image tools such as DALL.E, Midjourney, and others. The internet has been flooded with AI-generated images. These AI-based image generators use natural language to bring your imagination to life and beyond. But, the question is, are these tools creative enough?
Even with tiny horses, Deep Learning is just too primitive to let a horse ride on an astronaut! pic.twitter.com/9n2utUAWat
— Joscha Bach (@Plinz) May 24, 2022
Getting your image the way you want it can sometimes be frustrating. But that is also getting better with each passing day. For instance, DALL.E 2 (inspired by Salvador Dalí and WALL-E) uses a ‘diffusion model.’ The diffusion model helps encode the entire text into one description to generate an image.
But, sometimes, the text can have many more details, making it hard for a single description to capture it all. While they are highly flexible, they sometimes struggle to understand the composition of certain concepts, where it confuses the attributes or relations between different objects.
Researchers from MIT CSAIL (Computer Science and Artificial Intelligence Laboratory) have found a better way to make DALL.E 2 more creative.
In an interaction with Analytics India Magazine, MIT researchers said that they structured the typical model from a different angle to generate more complex images with better understanding. The team said they added a series of models together, where they all cooperate to generate desired images capturing multiple different aspects as requested by the input text or labels.
“To create an image with two components, say, described by two sentences of description, each model would tackle a particular component of the image,” explained the researchers.
Mark Chen, co-creator of DALLE.2 and research scientist at OpenAI, said that this research proposes a new method for comprising concepts in text-to-image generation not by concatenating them to form a prompt but rather by computing scores with respect to each concept and composing them using conjunction and negation operators.
Further, he said that this is a nice idea that leverages the energy-based interpretation of diffusion models so that old ideas around compositionality using energy-based models can be applied. “The approach is also able to make use of classifier-free guidance, and it is surprising to see that it outperforms the GLIDE baseline on various compositional benchmarks and can qualitatively produce very different types of image generations,” said Chen.
Bryan Russel, a research scientist at Adobe Systems, said that humans could compose scenes including different elements in myriad ways, but this task is challenging for computers. “This work proposes an elegant formulation that explicitly composes a set of diffusion models to generate an image given a complex natural language prompts,” he added.
How does it work?
The magical models behind image generation tools work by suggesting interactive refinement steps to get the desired image or output. It typically starts with a ‘bad’ picture and then gradually refines it until it becomes the selected image. MIT Researchers suggested that by composing multiple models together, they jointly refine the appearance at each step, so the result is an image that exhibits all the attributes of each model. “By having multiple models cooperate, you can get much more creative combinations in the generated images,” explained the researchers.
For instance, let us say you have a red truck and a green house. The model would confuse the concepts of the red truck and green house when these sentences get very complicated. In this instance, DALL.E 2 might make a green truck and a red house to swap these colours around. “Our approach can handle this type of binding of attributes with objects, and especially when there are multiple sets of things, it can handle each object more accurately,” claimed MIT researchers.
Shuang Li, a PhD student at MIT, said that DALL.E 2 could effectively model object positions and relational descriptions, which is challenging for existing image generation models. She said that the model is good at generating realistic images but sometimes has difficulty understanding object relations.
Further, she said that people could use their model for teaching beyond art and creativity. “If you want to tell a child to put a cube on top of a sphere, and if we say this in languages, it might be hard for them to understand. But our model can generate the image and show them,” Shuang Li added.
Making DALL.E proud
In the research paper ‘Compositional Visual Generation with Composable Diffusion Models’, the team’s model uses different diffusion models alongside compositional operators to combine text descriptions without further training. The team includes Li, Yilun Du, and Nan Liu, alongside MIT professors Antonio Torralba and Joshua B. Tenenbaum.
The research has been supported by Raytheon BBN Technologies Corporation, Mitsubishi Electric Research Laboratory (MERL) and DEVCOM Army Research Laboratory.
Next month, the team will present the work at the 2022 European Conference on Computer Vision in Tel Aviv.
The team’s approach captures the text more accurately than the original diffusion model, which directly encodes the words as a single long sentence.
For instance, ‘a pink sky and a blue mountain in the horizon’ and ‘cherry blossoms in front of the mountain’, the team’s model could produce that image exactly. In contrast, the original diffusion model made the sky blue and everything in front of the mountains pink.
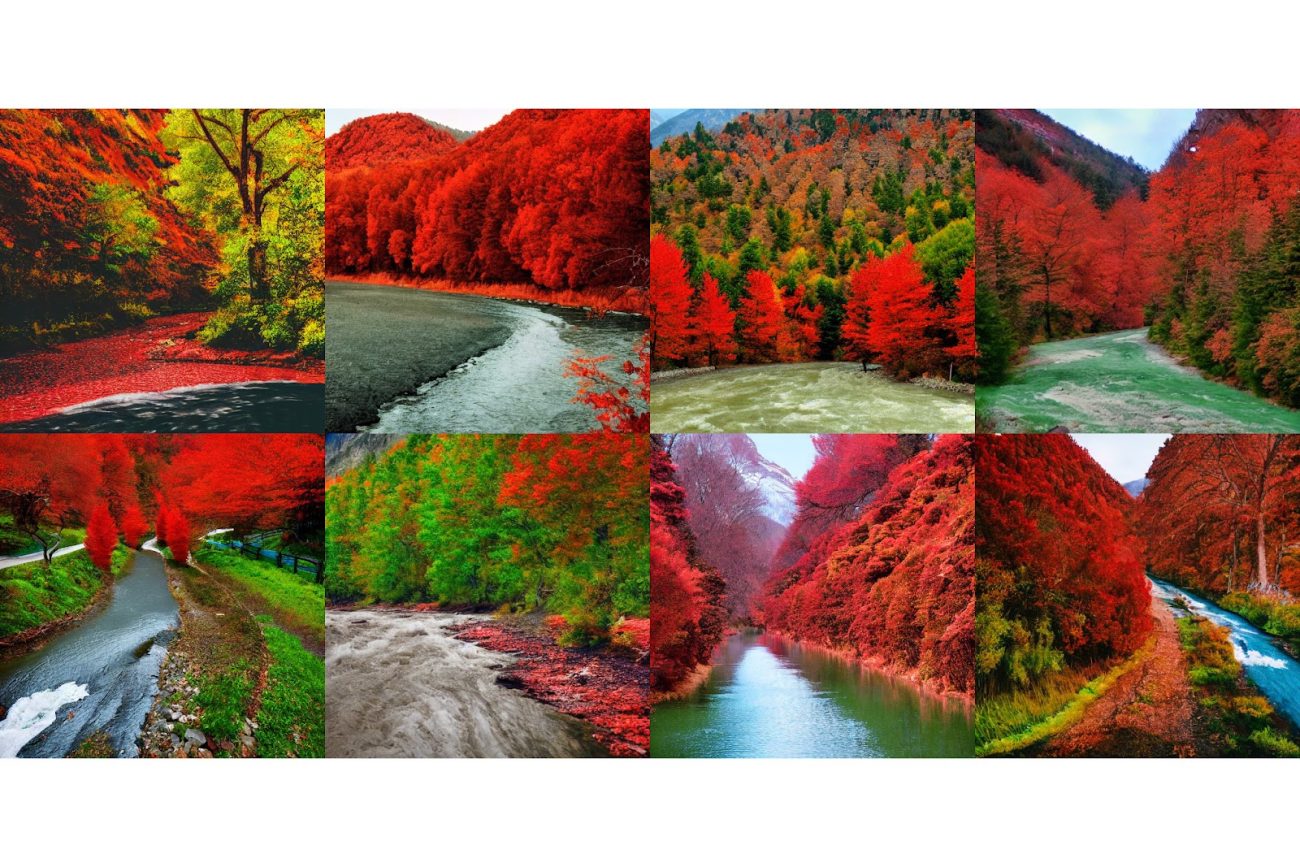
Du said their model is ‘composable’, meaning it can learn different portions of the models simultaneously. He said that it first leads an object on top of another, then learns an object to the right of another, and learns something left of another.
Further, Du said their system enables them to learn the language, relations, or knowledge incrementally, which they think is a pretty interesting direction for future work.
Limitations and opportunities
Even though their model showed prowess in generating complex, photorealistic images, it still faced challenges as the model was trained on a much smaller dataset than those like DALL.E 2. “So, there were some objects it simply couldn’t capture,” said the MIT researchers.
The researchers believe their ‘Composable Diffusion’ works on top of generative models like DALL.E 2. They want to explore continual learning as a potential next step. The team said they want to see if diffusion models can start to learn without forgetting previously learned knowledge—to a place where the model can produce images with the previous and new knowledge.