
What is moving? The man with the glasses? Or is the world collapsing around him? Actually, it’s neither — it is the camera that is moving inwards in a technique popularly-known as dolly shot. This distorting spacetime effect was first effectively used in Hitchcock’s masterpiece Vertigo (1958) to manipulate the attention of the audience.
Even with all the knowledge of the real world, it is tricky for humans to identify how far or close the objects in the videos really are — such as in the video above. It gets even messier when you task a machine to recognise the depth in the videos. Moreover, unlike the movies, the real world video streams can have objects/people moving along with the camera and other such possibilities.
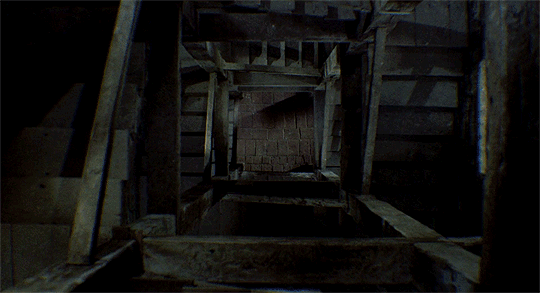
The existing 3D reconstruction algorithms will find such freely moving targets confusing. So, most existing methods either filter out moving objects (assigning them “zero” depth values) or ignore them (resulting in incorrect depth values).
A paper titled, Learning the Depths of Moving People by Watching Frozen People, published by the researchers at Google, talks about how a deep learning approach can be used to generate depth maps from an ordinary video that can tackle the previously mentioned challenges. This model avoids direct 3D triangulation by learning priors on human pose and shape from data.
This work is the first of its kind as it uses a learning-based approach to the case of simultaneous camera and human motion. The success of this method can find its significance in applications involving augmented reality and 3D video effects.
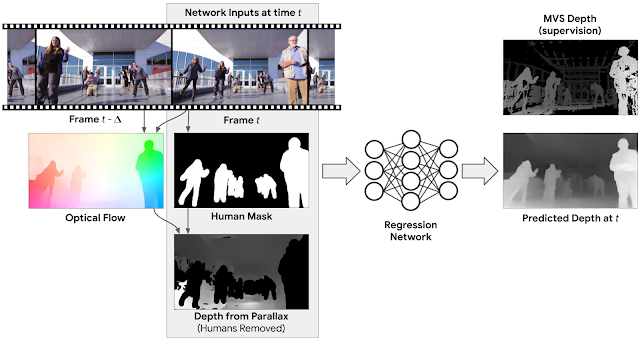
The objective here is to generate depth maps of videos having moving people and moving camera. For this, the authors pursue a multiple point approach where the motion of static point between two points can give how far or close a certain object is, in a frame.
To make sense from these multi-viewpoint cues, a 2D optical flow is calculated. Optical flow is a mathematical approach to identify the motion of an object in a frame. This was originally modeled around how animals perceive their surroundings as they move.
So, an optical flow would give out the difference between frames by considering pixel intensities and other such attributes. For instance, if an object is getting brighter with every frame then it can be inferred that the object is not only moving but also coming closer as well.
In this case, the camera position is predetermined, hence their dependencies are not considered. Images devoid of dependencies are fed and are checked for humans. The algorithm masks all the potential humans in the image using mask R-CNN. These masked regions are removed and this image is run through regression network, which predicts depth.
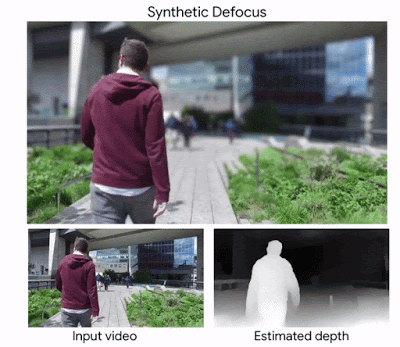
One cool outcome of generating depth maps algorithm is the synthetic defocus as can be seen above. As the network applies segmentation and optical flow computation, the target objects can be pushed out of attention and make other CG works in post-production tasks in case of movie making.
Even though this approach looks promising, there is still room for improvement. In videos where camera position is of less significance or unknown can trick the model. And, there are obviously non-human objects in almost all videos. However this approach will act as a vantage point for future works, which use neural networks to decipher and design videos.
Know more about this work here
Also watch: