The COVID-19 outbreak has engulfed the world and has been declared a global pandemic, four months since the first case was discovered in Wuhan, China. Early testing is key to manage any widespread communicable disease. That leads to quick identification of positive cases, quick treatment of infected individuals and immediate isolation to prevent spread. It also aids in the early and effective tracing of those individuals who came in contact with infected individuals, thereby abating any further spread. An aggressive testing strategy also helps diminish the mortality rate as a treatment in the early phase of disease significantly decreases the likelihood of the need for intensive care.
As per Worldometer, the number of confirmed cases globally (as of 01:45 IST on 11th April) stands at a staggering 16,85,610. For those countries (barring China) in which the total confirmed cases reported till date are greater than 1000, every 8th test has resulted in a confirmed case. This ratio is significantly bigger for few countries like UAE (~176), Russia (~91), Australia (~55), South Korea (~48) and New-Zealand (~47). These are also the countries which have optimally contained the disease spread and have been able to keep the mortality rate extremely low at 1.12% compared to the global rate of 6.1%. The other metric which is a major indicator of country level testing capacity is Tests/1M population. The countries with higher tests/1M population have been able to efficiently enable ample isolation and tracking and have been able to keep the mortality rate low.
In India, as per ICMR, a total of 1,61,330 samples from 1,47,034 individuals have been tested as on 10 April 2020, 9 PM IST. 6872 individuals have been confirmed positive among suspected cases and contacts of known positive cases in India. The metric Tests/1M population for India is 137, which is pretty dismal in comparison to average of 9,882 in those countries where the number of confirmed reported cases are greater than 1000. Some of the countries across the globe which have managed to control fatalities from COVID-19, such as Germany and South Korea, have one thing in common: they tested a lot. India’s testing rate is one of the lowest amongst those countries which have had more than thousand confirmed cases till date.

An analysis on historical data indicates a spurt in number of confirmed cases as the rate of testing increased in India. The states which have more testing centres and are performing more tests, have reported a higher number of coronavirus cases. India’s testing strategy is confined to testing those individuals who are symptomatic and have some travel history or have come in contact with corona positive individuals. One of the key reasons behind lower testing rate is the lack of vital testing infrastructure and the cost associated with the tests. There is an impending need to adopt an aggressive testing strategy and test more individuals – both symptomatic and asymptomatic.
The biggest constrictions around increased testing capacity is lack of testing kits and lack of healthcare individuals administering these tests. In this treatise, I propose a quantitative framework to increase the testing capacity within the constraints of infrastructure, resources and workloads.
The Framework
One of the easiest and most efficient method to increase the testing capacity multifold is by conducting pooled testing i.e. putting multiple swab samples together and testing them using a single RTPCR test. If that test is negative, it means that all the people tested are negative. However, if the test is positive, everyone whose samples were part of the positive pool have to be tested individually. In this framework, I propose to mix 2n samples together for pool testing and in case of a positive outcome, use binary search for identifying the positives.
The study proposes a novel approach to determine ‘n’ based on historical global and domestic COVID-19 testing data.
Determining the value of ‘n’
In India, the testing has been confined to symptomatic individuals with travel history and asymptomatic or mildly symptomatic contacts of COVID-19 confirmed cases. The current stats indicate that every 24th test in India results in positive. One of the key factors which determines the efficacy of mitigation measures at a country level is the rate of mortality which is defined as the ratio of total deaths to total confirmed cases. The global mortality rate currently is at ~6.1% with 13 countries above the global average. India’s mortality rate is ~3.1% and amongst the countries with more than thousand cases, 36 countries fare better than India in terms of mortality rate. A mortality rate of less than 1% is a testimonial to robust containment and mitigation strategy and can be attributed to widespread testing of asymptomatic and unsuspected cases.
The value of ‘n’ has to be a function of the likelihood (propinquity) of the individual being positive. The propinquity has to be determined based on factors like exposure or close contact with COVID-19 infected individual, travel history or exposure to COVID-19 hotspots, COVID-19 symptoms like fever, dry cough, breathlessness etc. Historically, only high propinquity individuals have been tested in India and the ratio of positive to negative cases have been 1:23. In countries, where low propinquity random tests have been conducted, the ratio of positive to negative cases remains at 1:70*.
*Countries Considered – UAE, Hong Kong, Russia, Bahrain, Australia, New Zealand & Singapore
Scenario 1: High Propinquity Samples:
Based on the ratio of positive to negative cases in India, the optimum value of ‘n’ will satisfy the below inequation:
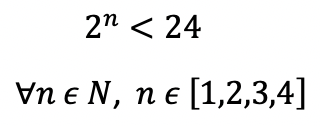
Hence, ‘n’ can range from 1 to 4.
Based on historical data, the probability of a sample to be positive for high propinquity samples:
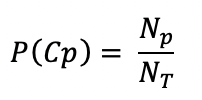
Where;
- Positive Case
- Total Positive Cases
- Total tested Samples
Considering India’s sample space as high propinquity, the probability of a sample being positive is 0.0423 (7,997 positive cases out of 1,89,111 samples).
Assuming that there is no repeated test for an individual and the outcome of any test does not depend on the outcomes of any other tests and that all tests are identical, each test can be considered as a Bernoulli trial. Sequence of ‘t’ such trials will follow a binomial distribution.
Random variable ‘X’ is the number of successes (positive cases) that occur in the ‘t’ trials (tests).
Distribution:

If n is 1, 21 = 2 samples will be tested together. According to Binomial theorem, for n=1, the probability that at least 1 sample is positive given the probability of a sample being positive is 0.0423 will be:

For n=1, the probability of at least one sample being positive is 8.2%. Similarly, for n = 2, 3 and 4, the probability of at least one sample being positive is 15.9%, 29.2% and 49.9% respectively. The likelihood of at least one sample being positive when 16 samples are tested together is still less than 50%.
For a given value of ‘n’, the maximum tests which would be required to be done will be 2n+1-1. Hence, for n= 4 (pool size = 16), the maximum number of tests required to be done would be 31.
If 1000 pool tests of 16 samples each are conducted and the pools containing positive samples require 31 tests (worst case scenario), the minimum number of pools (p) having all negative samples such that the pool testing approach requires lesser tests will be 500 (calculation below).

The probability that a pool will have at least one positive sample is 0.499. Hence the probability that at least 500 out of 1000 pools will have positive samples:

= 0.487 [Binomial Approximation]
The likelihood of at least 500 pools having positive sample is less than half. Hence, it can be safely concluded that a pool size of 16 samples will be optimum for testing high propinquity cases.
Scenario 2: Low Propinquity Samples:
Based on the ratio of positive to negative cases for low propinquity samples, the optimum value of ‘n’ will satisfy the below inequation:
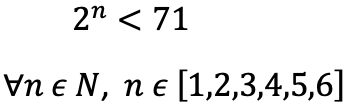
Hence, ‘n’ can range from 1 to 6.
The probability of a low propinquity sample to be positive is 0.014 (42,558 positive cases out of 30,35,923 samples.
According to Binomial theorem, for n=1, the probability that at least 1 sample is positive given the probability of a sample being positive is 0.014 will be:

For n=1, the probability of at least one sample being positive is 2.7%. Similarly, for n = 2, 3, 4, 5 and 6, the probability of at least one sample being positive is 5.4%, 10.6%, 20.2%, 36.3% and 59.4% respectively. The likelihood of at least one sample being positive when 32 samples are tested together is still less than 50%. However, if 64 samples are tested together, the likelihood of the pool being positive is ~60%. Evaluating this further empirically for 1000 pools with 32 samples in each pool and 64 samples in each pool such that at least 500 out of 1000 pools have positive samples gives probability of 0.0000000000000000012 and 0.9999999992 respectively indicating that while the first event is almost uncertain, the second one is almost certain. Since the probability score of 0.014 of a sample being positive is extremely low and the best globally, a pool size of 32 will suffice even for a higher probability score of 0.02 with the likelihood of at least 500 positive pools out of 1000 pools being ~7%.
Hence, it can be safely concluded that a pool size of 32 samples will be optimum for testing low propinquity cases.
The Binary Search Consideration
The approach of pool testing and employing binary search in case of positive result will enhance the testing capacity multifold.
For a pool size of 2n, the worst-case scenario will mean a total of 2n+1-1 tests for a given pool. Considering a pool size of 16 samples, the worst-case scenario would be when each node in the tree would be tested because of at least one positive sample within it. If there is a presence of positive samples in the pool, the minimum tests required would be 9 and the maximum tests required would be 31. Let Ƭ be the number of tests required for a pool given that the pool has at least 1 positive sample. Ƭ will follow an arithmetic progression with common difference of 2. Ƭ can take any value in this range with varying probability.

In the above representation, there is a possibility of 1 or 2 positive samples and the positive sample(s) always fall(s) in the right node at each level. This is the best-case scenario given that at least one positive sample is there in the pool and the number of total tests required in the case would be 9.
A linear search scans one item at a time without skipping any of the items. However, in case of binary search the search space is cut down to half as soon as the middle of the sorted list is discovered. Hence, binary search is more efficient with a time complexity of O(log p) compared to O(p) in case of linear search ; p being the pool size.
Conclusion
Pool testing with binary search is an efficient method to enhance countrywide testing capacity. A pool size of 16 for high propinquity samples and a pool size of 32 for low propinquity samples is recommended considering the historical trend and statistics from other countries. The adoption of the proposed technique will significantly increase the testing coverage and will also reduce the time and effort required for tests per million of population. The efficacy of the proposed technique can be evaluated by adopting it for a subset of testing labs and evaluating the metric # of individuals tested per testing kit.