Listen to this story
|
The concept of synthetic data is almost too good to be true – it can mimic the distinctive properties of a dataset while dodging a number of issues that afflict data. There are zero data privacy concerns around synthetic data since it is artificially generated and isn’t related to real-world persons. It can be manufactured on demand and in the volumes required. In other words, synthetic data is a boon in a world eternally thirsty for data.
And the hectic space of generative AI is offering a helping hand in the easy generation of synthetic data.
The concept of synthetic data has been around for decades until the autonomous vehicle (AV) industry started using it commercially in the mid-2010s. But for how important an issue it resolves, creating synthetic data brings a myriad of complications along with it.
At times, synthetic data was harder for companies to afford because it used to run on high-end generative models that were normally expensive. The hardware required was heavy on the pocket and had to be supervised by data scientists. They also had to be tried and tested multiple times to generate the final, tailormade output.
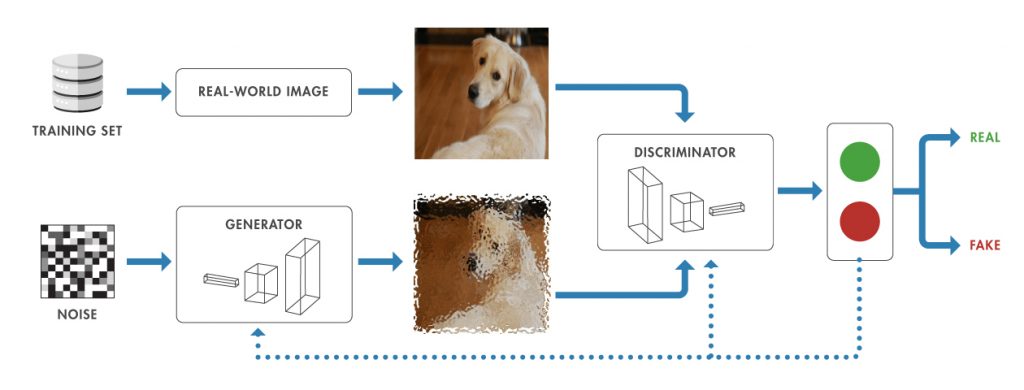
Problems of making synthetic data with GANs
Until now, GANs (Generative Adversarial Networks) were considered to be reasonably good for producing realistic images. But then again, they weren’t necessarily the most efficient – GANs were great while generating new faces from datasets of faces but normally broke down when used in very broad visual areas like the AV driving sector.
Being an early iteration of generative models, GANs have other hiccups. Their network structures had to be specially adapted to process specific data formats (images or tabular data). They were also considered poor at including potential outliers or unusual data points in their model.
Also, the training process under GANs can be a little tricky. It can be challenging to find the correct hyperparameters and there is no easy way to judge when GANs have been trained to an appropriate measure. GANs function in a way where the generator or the discriminator learns a new trick raising the adversary’s loss again. This means that the back and forth between the two adversaries during training also keeps adding to the total compute.
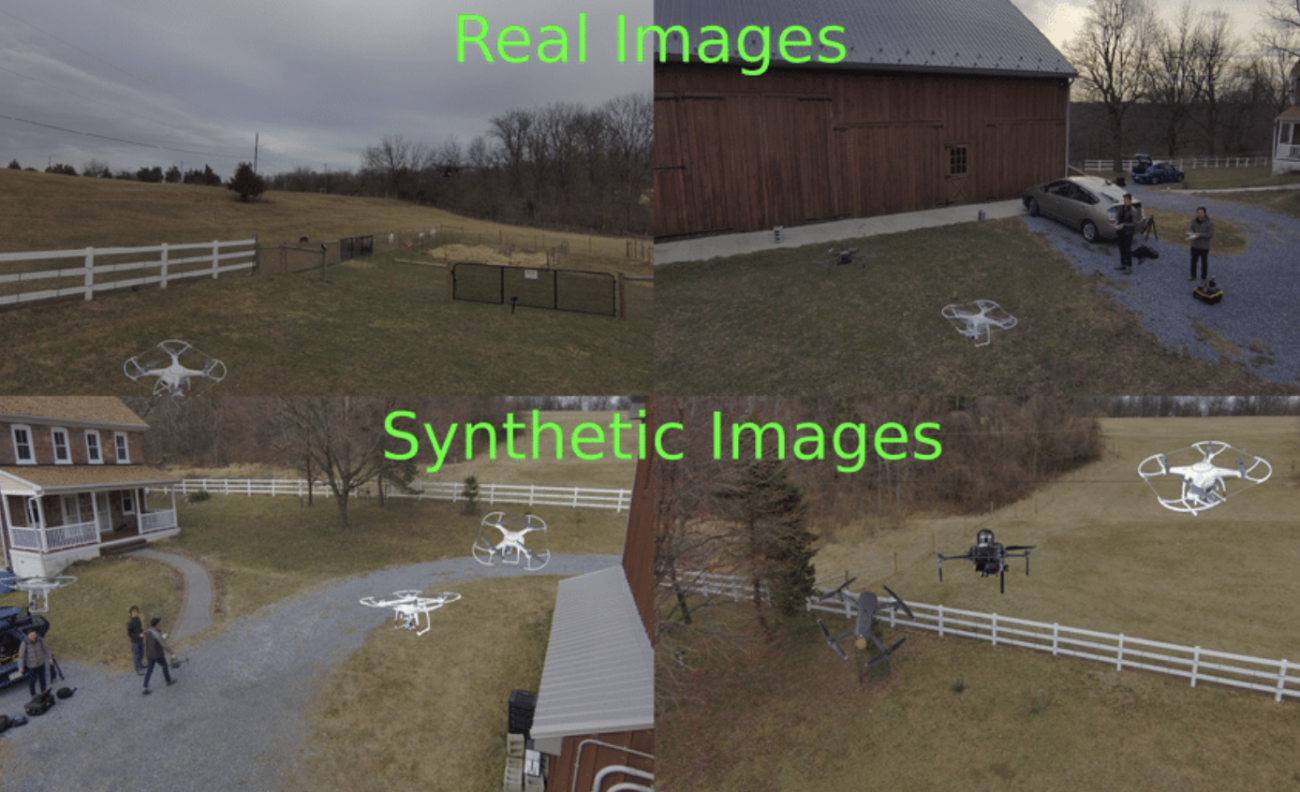
Text-to-Image generators for synthetic data creation
But the new age generative AI text-to-image models like DALL.E, Midjourney and Stable Diffusion are some of the simplest versions of this range of generative tools. All these tools have been built on diffusion models which encode text into a compressed state and then use a decoder to convert this representation into an image. The proof of their easy usage is how mainstream these tools became in little time – they were quick, basic and used relatively lesser compute while giving drastically better results.
At the MLDS conference held this year, Aditya Bhashkar, computer vision engineer from iMerit discussed how he had experimented building synthetic datasets with Stable Diffusion. The results were presented in a paper titled, ‘Leveraging Machine Learning and Computer Vision Strategies for High-quality Data Augmentation and Synthesis in AgTech’, that Bhashkar co-authored with Ranti Dev Sharma, Divakar Roy, Saravanan Murugan, Anubhav Srivastava and Aparna Prabhu.
When asked if there were any uphill tasks while using generative AI tools, Bhashkar admitted that fine-tuning these models just in accordance with their specific use still took some efforts. But fine-tuning the different iterations of these generator and diffusion models would regardless need a lot of compute.
However, he continues to see practical applications of these tools as they evolved in this sphere. “Till now, we have been seeing tools very specific to the use case. But, over the past few years, new tools and architectures that have the capability to generalise well to various use cases have come up, reducing the requirement for fine-tuning for every use case,” he added.
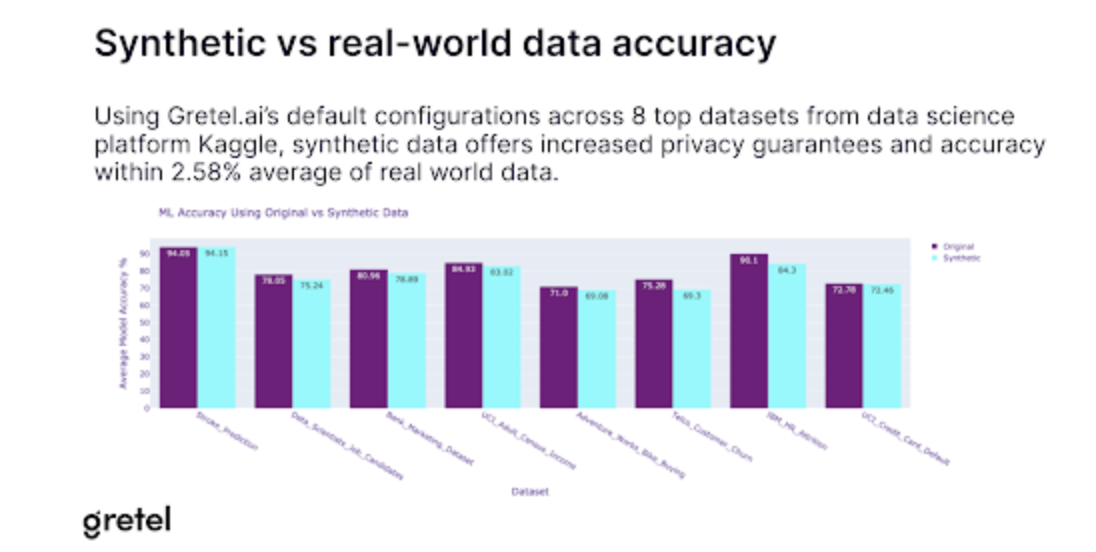
Synthetic data everywhere
Companies have spent far too much time collecting, labelling, training and deploying data and datasets, which doesn’t just weigh heavy on companies’ pockets but also eats into time. AI teams have been found to be shelling out anywhere between 50% and 80% of their time collecting and cleaning data while spending around USD 2.3 million a year just on data labelling.
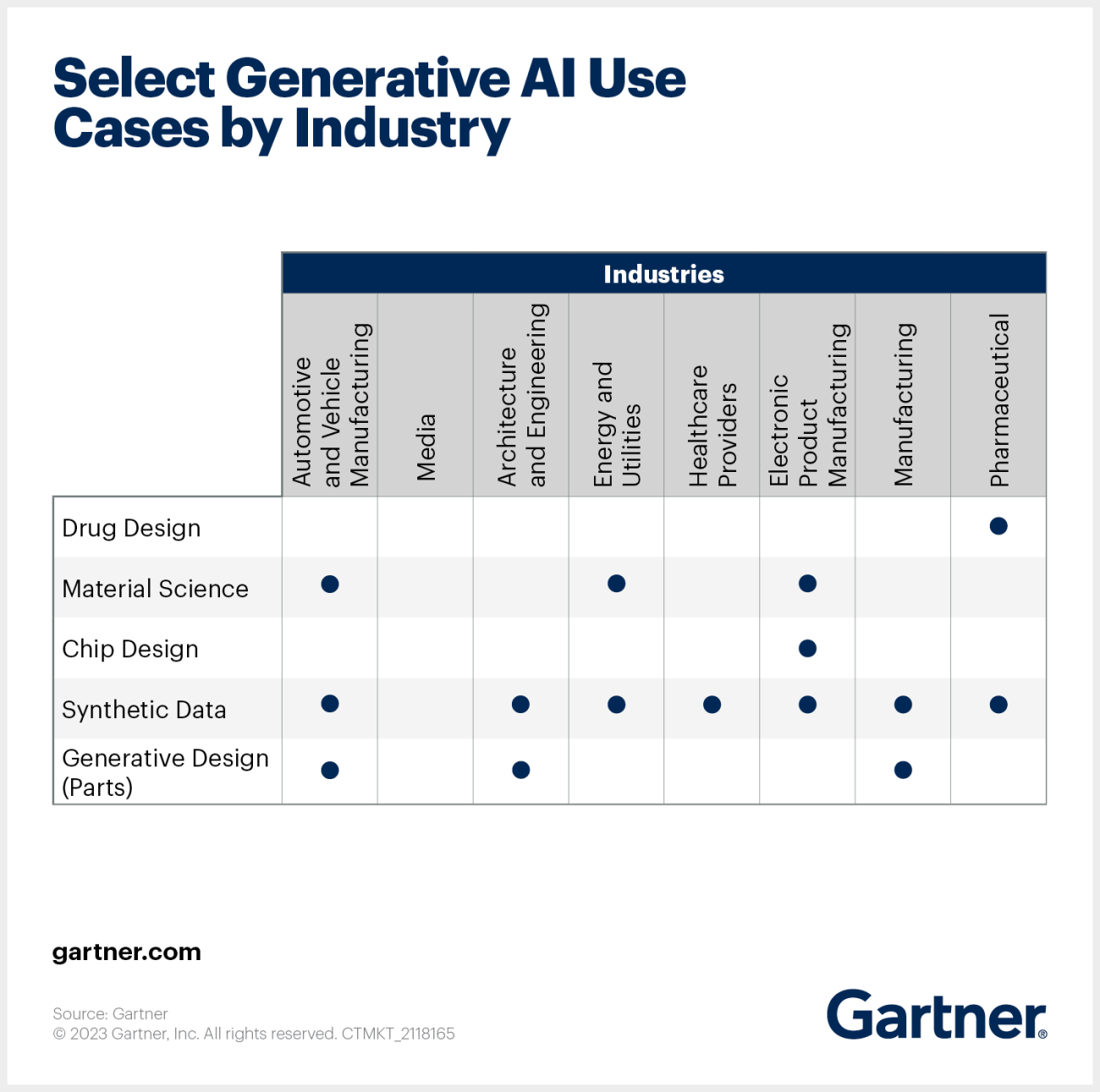
Under these conditions, it becomes natural for companies to shift towards synthetic data. In fact, a Gartner study that has been heavily referenced throughout stated that by 2024, 60% of all data in AI would be synthetic.
An article by Andrey Shtylenko around use of generative tools for creating datasets, Yashar Behzadi, CEO and founder of Synthesis AI welcomed the era of these compact generative AI models saying, “With the advent of diffusion models trained on such large visual datasets, domain adaptation will now be possible even with the widest visual domains. This changes the game for synthetic data allowing us to create data in simulation and transform it to completely realistic output. This will also transform video games and enable a new level of generative synthetic media while leveraging very similar technology.”